Enchant whitepaper: Breaking the data wall between lab and clinic
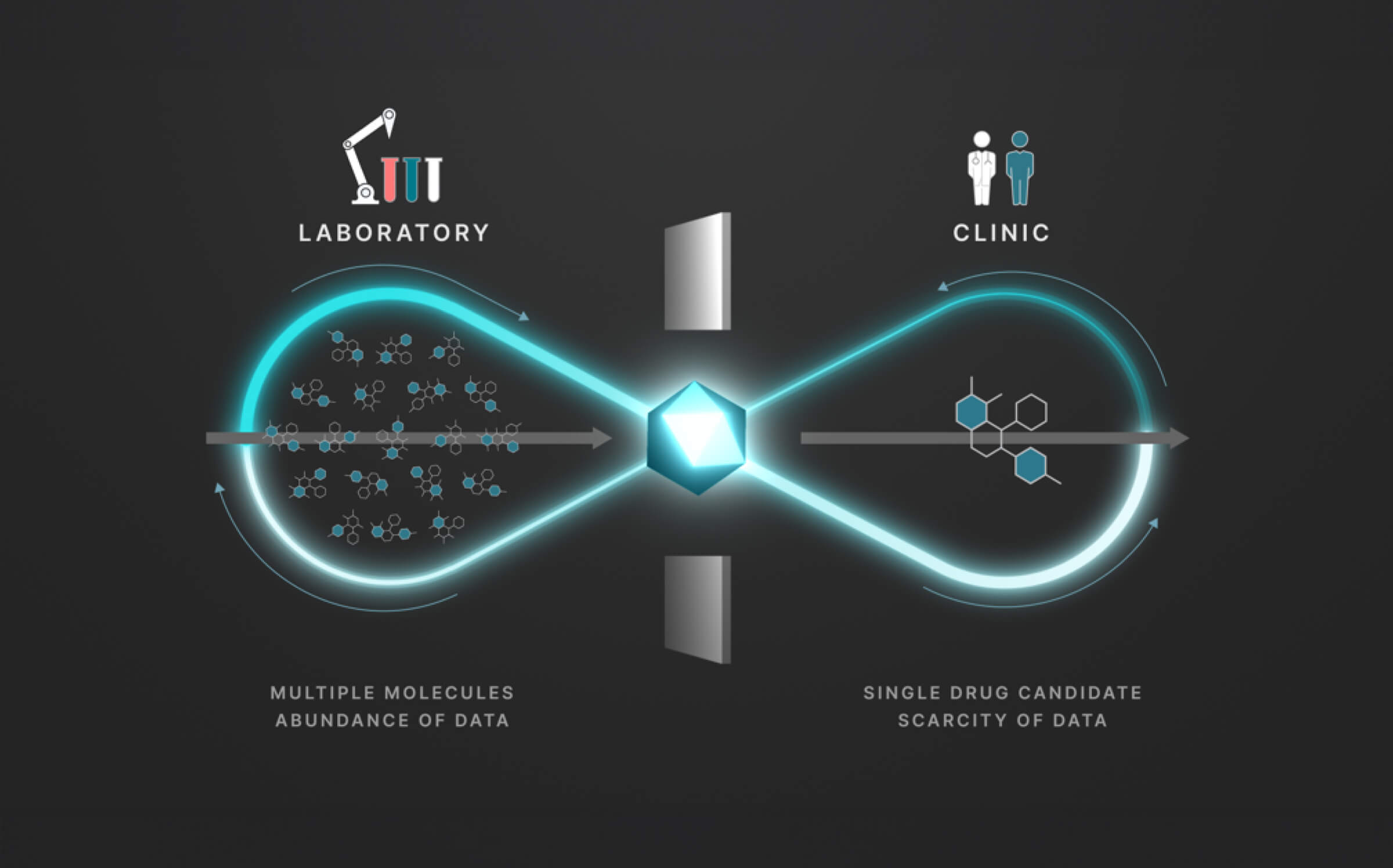
Enchant is a breakthrough multi-modal transformer trained on dozens of data modalities and sources from across the full span of drug discovery and development.
The discovery and development of new medicines is inefficient and expensive, leaving many patients' medical needs unmet. Sector-wide, low efficiency is driven by late-stage clinical failure.
The full process involves two distinct stages: a discovery stage, where many (for example thousands of) molecules are under investigation in the laboratory; and a clinical stage, where a single drug candidate is tested in human volunteers or patients.
Innovations in artificial intelligence have improved and accelerated many aspects of these stages individually. For example, impressive progress has been achieved in target identification, molecular discovery, and clinical trial design.
Data from the discovery stage is abundant, and becoming even more abundant through laboratory automation. But economic and ethical barriers prevent generation of clinical data on any but a vanishingly small number of distinct molecules. This creates a data wall between the laboratory and the clinic, and is the fundamental obstacle to the efficient creation of medicines.
At Iambic Therapeutics we have developed Enchant, a cutting-edge AI model, that breaks through this wall.

The purpose of Enchant is to make predictions for data-scarce clinical outcomes by leveraging abundant discovery data. It builds on our previous work, which addressed two challenges: different stages create data of different types, and public data sources are messy and of mixed quality. Here we use Enchant to predict clinical properties by training on increasing amounts of discovery data.
As a first demonstration, we predict human pharmacokinetics (PK) of candidate drugs. We organize the discovery data in the order that they are typically gathered during the discovery process. Enchant produces reliable predictions of clinical PK properties by being trained on more pre-clinical, laboratory data, as shown in Figure 2. We see that training Enchant with clinical data on just 5 distinct molecules (less than 1% of the dataset) yields strong predictive power. Competitor technologies trained at such low training set sizes do not yield predictive models.
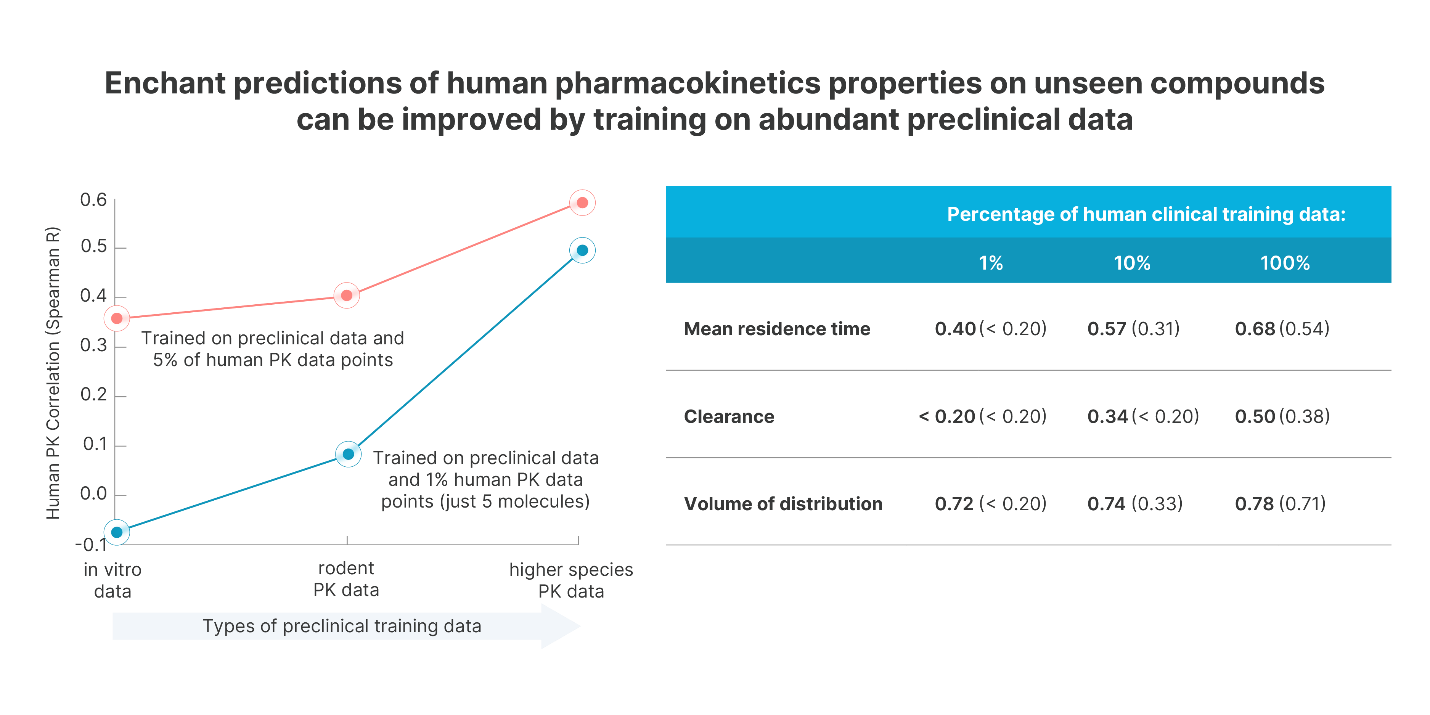
Enchant also surpasses the state of the art when all clinical PK training data are used. Trained on all of the available preclinical data together with the full Obach dataset, Enchant achieves a Spearman R of 0.74 for human PK half life, outperforming the previous state-of-the-art performance of 0.58. Similar performance is observed for other human clinical PK properties.
While Enchant represents a paradigm shift in breaking the data wall between laboratory and clinical data, it's important to contextualize its performance within the landscape of existing models (Figure 3). Several groups have attempted the development of large language models (LLMs) for drug discovery, but none approach the predictive power of Enchant, and Enchant's ability to leverage abundant pre-clinical data to make accurate clinical predictions is a unique and fundamental breakthrough in the field.

Drug development is inefficient and costly, mainly due to the lack of clinical data, which leads to late-stage failures. Enchant, our new AI model, breaks the data wall by using abundant discovery data to predict clinical outcomes. By additionally incorporating even small amounts of clinical data, it outperforms existing models trained on full clinical datasets.
Enchant has enabled Iambic to reduce clinical risk in our programs at the discovery stage. Even in the absence of clinical data, it provides valuable insights to avoid potential pitfalls. Combined with our other AI and platform technologies, Iambic works to efficiently deliver safer, more effective medicines.
Contact: partnerships@iambic.ai
1 We’re primarily addressing small molecules, but similar considerations apply to other therapeutic modalities.